The industrial vision product team of the artificial intelligence company Innovusion, relying on the ManuVision industrial vision platform, has accumulated valuable experience in empowering with AI technology and implementing products in multiple typical scenarios of industrial manufacturing. This article, in the form of a Q&A, discusses with the head of the industrial vision products at Innovusion the practical challenges faced by the implementation of AI in the field of industrial vision, as well as the core methodology and technological product strategies adopted by the Innovusion team to address these challenges.
Author | The Industrial Vision Product Team of Innovusion
Question: What core values can cutting-edge technologies represented by AI contribute in industrial manufacturing, especially in industrial vision scenarios?
Answer: Industrial vision is a core area of industrial automation, encompassing key tasks such as inspection, recognition, measurement, and positioning. AI technology, which excels in solving visual perception problems, has become an important entry point for creating value. Today, the global manufacturing industry faces enormous pressure for industrial upgrading. Except for a few leading large-scale production enterprises at the top, most manufacturing production lines in the industry are confronted with the contradiction between the rapid iteration of demand and the insufficiency of informatization, automation, and flexibility: On the one hand, the rapidly changing global market calls for efficient production lines that can produce, customize, and iterate according to demand. On the other hand, practical problems such as difficulties in data collection and connectivity, low degree of automation, lack of coordination between automated workstations, and the difficulty in quantitatively evaluating the yield rate and accurately attributing its causes together pose an overall challenge to the improvement of manufacturing efficiency.
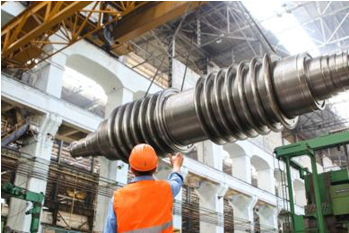
Most manufacturing enterprises need to make great strides to fill the gaps in informatization, automation, and intelligence within a relatively short construction period and achieve leapfrog development. Cutting-edge technologies such as the Internet of Things, big data, machine vision, automatic planning and decision-making, and automatic control are key technologies in this process. Among them, AI-related technologies are the foundation for the leap from "manufacturing" to "intelligent manufacturing".
Question: Specifically in industrial vision scenarios, why is artificial intelligence needed in industrial vision?
Industrial vision aims to solve the problems of how to "see" the manufacturing scenarios clearly and how to "perceive" and "understand" the key information. Currently, significant progress has been made in optical imaging technology, multi-sensor fusion technology, and photosensitive and optical processing chip technology, making it possible to "see smaller and more clearly". On this basis, AI can precisely help us "perceive more accurately and understand more deeply".
Take the electronics manufacturing industry as an example. The annual labor cost of the workstations related to visual inspection on the relevant production lines in China is about 6 billion yuan. The introduction of cutting-edge technologies will upgrade the visual inspection process from a relatively rough, difficult-to-quantify, and labor-intensive workstation to a fully automatic workstation that can be accurately quantified, fully traced, and intelligently integrated with data, resulting in a significant improvement in production efficiency and product quality.
Question: What kind of experience is it to plan, define, and design a product or solution in the field of industrial vision?
The challenges are huge. If we talk about the experience, it's like "suffering the mind and toiling the body". In the field of industrial vision, the forward development of a product goes through several stages such as demand research, overall scheme design, key technology verification, subsystem scheme design, prototype trial production, on-site scheme verification, product release, and market promotion. At each stage, the product manager needs to lead the team to polish and optimize with all their efforts in the right direction.
In the above process, the product manager's thinking needs to switch between the macro and the micro at any time: macro thinking is required when considering customer needs and product positioning; when considering the technical implementation, it is necessary to focus on the technical details at each key level; when laying out the product line, it is necessary to consider the matching of differentiated configurations for various types of customers; when verifying the on-site scheme, it is necessary to pay attention to specific data and customized requirements.
However, being a product manager in this field is also full of a sense of achievement: industrial vision products, especially automated visual equipment, are the products that can most bring a sense of achievement. When the product is successfully delivered and operates stably on the customer's production line, the customer's recognition is the best reward for the product development team.
Question: In this field, what are the product laws different from those in other fields?
First of all, there are many demand items: In the field of industrial manufacturing, the two indicators that customers care about most are production capacity and quality. Around these two seemingly contradictory indicators, the product design of industrial vision can be disassembled into multiple design indicators:
The main indicators related to production capacity include the handover mode between upstream and downstream, the TT (tack time) of products/equipment/instruments, product stability (MTBF, MTBR), machine type switching time, etc.
The main indicators related to quality include inspection/measurement accuracy rate, false detection and missed detection, repeatability, etc.
Due to the special environment of the industrial site (constant temperature, constant humidity, ultra-clean, safe), the main indicators to be considered include the ESD, weight, emergency stop, FFU, etc. of the product.
Customers in the industry will provide detailed specification requirements during the scheme communication stage, sometimes even covering hundreds to thousands of specification definitions.
Secondly, there are many sources of demand: Products in the industrial manufacturing field have distinct characteristics of enterprise-level (ToB) products, and the decision-making party, the purchasing party, and the using party are usually not the same team. For example, the decision-making party may be the engineering technology department or the equipment manufacturing department, the purchasing party is the procurement department, and the using party is the production department. Different customer departments are faced at different stages, and different departments have different demands for the product.
Thirdly, the degree of customization is high: Due to the different production line layouts, production line speeds, upstream and downstream process equipment, and even the different heights of elevators (determining the maximum height of the equipment) of different customers, the products delivered to each customer are highly customized. However, for the same industrial vision product series, its core functions should remain stable.
Question: Industrial vision technology can be abstracted into several aspects such as how to "see", how to "perceive/understand", how to "plan/decide", and how to "execute". Taking "seeing" as an example, what is the current level of relevant imaging technology, lighting technology, etc.?
From the perspective of the imaging chip dimension (more precise, better, wider, faster):
The improvement of the chip process technology has made large-format CMOS chips the mainstream chips for industrial cameras. The evolution from 2M→12M→29M→60M→71M→150M enables industrial inspection to reach the micron-level accuracy. The characteristics of high full-well capacity, high dynamic range, and low noise have greatly improved the imaging quality of industrial cameras;
The high-precision coating process has achieved pixel-level coating. Polarization cameras and hyperspectral cameras based on the above technologies can obtain more dimensional information of the products to be detected in industrial inspection scenarios;
The TDI technology can greatly reduce the exposure time and increase the scanning frequency of the camera. With the high-speed image capture card, visual inspection can be installed on the high-speed automated production line.
From the perspective of the lighting source dimension: Ten years ago, the lighting source products in the machine vision industry were basically monopolized by Japanese enterprises. Today, domestic machine vision lighting source manufacturers have risen rapidly, and the variety and quality of lighting source products have been continuously improved. Currently, common LED lighting sources (strip light, ring light, coaxial light, dome light, backlight) and lighting source controllers have been widely used in various visual systems. Domestic suppliers also actively cooperate with the lighting verification and lighting source schemes for various demands and scenarios. At the same time, domestic lighting source suppliers are actively promoting the research and development of independent lighting sources and even visual systems, such as multi-angle line light sources, line scan time-sharing exposure systems, visual integrated controllers, etc. In addition, projected structured light and laser line light sources have also been applied in a number of 3D contour or defect detection scenarios.
From the perspective of the imaging scheme dimension: Currently, there are various imaging schemes for industrial vision products and equipment. Multiple schemes such as area array schemes (stationary or flying shot detection), line array schemes, line laser scanning, coded structured light, white light confocal, etc. have been maturely applied. And complex multi-station inspection equipment often integrates the above multiple schemes to achieve higher imaging rate coverage and 3D contour measurement. It is believed that in the near future, new technologies such as computational imaging, hyperspectral imaging, and light field cameras will be further integrated into industrial vision schemes.
Question: How do "light" and "optics" affect the technical implementation of specific projects? What issues should be focused on in the design to make good use of optical technology in products?
As an optical engineer, the basic concept is "Garbage in, garbage out", which also shows the influence of the optical system on industrial vision projects.
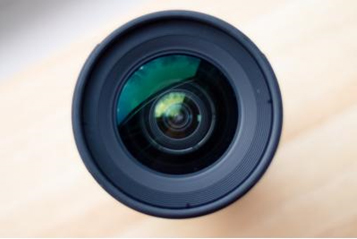
At the overall scheme level: Complex industrial vision equipment basically integrates multiple imaging schemes, and thus also has a multi-station design. Only when the optical scheme is determined can the station distribution and equipment layout of the equipment be determined.
The optical system imposes constraints on the structural and automation design of the product: Driven by demand, industrial vision products have high resolution. At the same time, DFX requirements such as the debuggability and maintainability of the equipment also need to be considered. The visual system usually needs to reserve an adjustment mechanism. A qualified optical engineer will, while designing the optical scheme, output the index constraints of the visual scheme on the machine vibration, positioning repeatability, adjustment freedom of the visual mechanism, adjustment range of each degree of freedom, flatness of the stage, etc. Only when these indicators are clear can the downstream design avoid rework and make debugging easier.
The optical system imposes index constraints on the algorithm performance: In the industrial inspection scenario, the core indicators of industrial vision inspection equipment are the defect detection rate and inspection accuracy rate, etc. For example, if the customer requires a defect detection rate of 90%, it can generally be disassembled into x% of the defect imaging rate multiplied by y% of the defect detection rate in the imaging. The product of the two indicators is about 90%, so each individual indicator needs to be much higher. When conducting imaging verification, the optical engineer needs to communicate closely with the algorithm engineer to confirm whether the defect imaging meets the requirements of algorithm detection, with the ultimate goal of ensuring the defect detection rate.
Overall, we believe that in the research, development and design of industrial vision products, optics must come first. In addition to designing the optical scheme, the vision engineer also needs to invest a lot of energy in research topics such as the pre-research of advanced imaging methods and advanced products. For example, in the LCD era, conduct pre-research on OLED products and processes, and in the OLED era, conduct pre-research on QLED and Micro LED. In this way, during the continuous 更迭 of upstream products and processes, the knowledge accumulation will not fall behind. In short, the vision engineer should, like the automation engineer - or even more so - understand the processes and technologies.
Question: At the "perception" level, to what extent do the industrial vision software and hardware components need to perceive the workstation scenarios to meet the business requirements?
Perception has multiple meanings: In the inspection scenario, the visual product accurately detects defects and replaces manual work quickly and well; in the alignment scenario, the visual product identifies the objects to be grasped/assembled/crimped, etc., and accurately feedbacks the position of the objects; in the measurement scenario, the visual product measures the geometric quantities that the process focuses on and feedbacks accurate measurement results. The above scenarios all require the reasonable selection, design and configuration of visual algorithms.
For the inspection scenario, the basis of perception needs to be established on the customer's manual inspection benchmark. For various products and processes to be inspected such as films, rolled materials, glass, etc., as long as there are manual inspection workstations, there will be specific and detailed manual inspection benchmarks. The first step is to detect the "suspected abnormalities" in the images through various algorithms/models (feature detection, object detection). After that, it is more important to understand the customer's manual judgment logic and design the corresponding algorithm logic. For example, common manual judgment benchmarks will specify the length, width, area, point group distance, depth, etc. of the defects, and our algorithm logic also needs to be designed based on this.
For the measurement scenario, common methods include 2D and 3D measurement. In this type of scenario, it is necessary to first confirm the inspection accuracy required by the customer, and then disassemble the key indicators such as the resolution of the visual system and the accuracy of the measurement algorithm. There was once a customer who used a two-dimensional (projection image measuring instrument) device as the benchmark measuring device. All measuring devices, if they pass the acceptance inspection, need to be compared with the measurement results of the two-dimensional device - in this case, even if the measurement result of a certain device has reached the limit of its measurement principle, if it does not match the benchmark device, it cannot be delivered smoothly.
The alignment scenario is more common in processes such as assembly, grasping, mounting, and drilling. This also requires first confirming the alignment accuracy required by the customer. The positioning accuracy required by the electronics manufacturing industry has reached the micron level. Each link such as the calibration, feature recognition, and coordinate calculation of the alignment visual components requires sub-pixel level accuracy, and in some scenarios, multiple alignments are required to ensure the accuracy.
Question: How should a good industrial vision product or solution select and combine different perception technologies?
Comprehensive ability is the key. Manufacturing customers are more inclined to assign the entire production line or the visual products in the entire production line to one solution provider/equipment manufacturer for integration. In this context, if you want to obtain higher-quality orders, you must, on the basis of specializing in one type of visual product, also have the control/design/development capabilities of other visual products or solutions. For a good industrial vision product or solution, it must have the ability to solve various visual scenarios completely. Based on traditional algorithms such as 2D/3D measurement and feature detection, and relying on detection, classification, and segmentation based on deep learning to create differentiation in some complex scenarios.
Question: Compared with traditional computer graphics and traditional computer vision technologies, what are the advantages of the new generation of AI technologies represented by deep learning? What is the relationship between deep learning technology and traditional technologies in solving industrial vision problems?
For example, in some appearance defect detection projects, only traditional algorithms were used in the early stage. Under the premise of ensuring the defect detection rate, the false detection rate was high, and the workload of manual re-judgment by customers was large, and it did not reduce much manpower for customers. After analyzing the false detection images, it was found that the false detections were mainly caused by dirt, dust, etc., and it was difficult for these false detection sources to be distinguished from real defects relying on traditional algorithms. By introducing deep learning algorithms, the effect of suppressing false detections based on deep learning classification was verified. After several rounds of model optimization (return of on-site false detection images → model training and model update → on-site verification and continued feedback of false detection images), the false detection rate was greatly reduced, and the customer was very satisfied.
Another example is that the appearance inspection of mobile phone finished products has always been a very difficult direction in the electronics manufacturing field. Few manufacturers dared to try before 2017. After 2017, with the diversification of the hardware schemes of visual devices (strobe, flying shot, 6-axis robot), some manufacturers gradually began to try in the market. Due to the large number of functional modules of mobile phones (camera, earpiece, speaker, buttons, charging port), diverse shapes (glass, metal, mirror surface, frosted surface, chamfer, curved surface), and diverse types of defects (a comprehensive scenario of all appearance defects), the imaging situation is complex, and it is difficult to cover all defects with traditional algorithms based on feature detection. Algorithms based on deep learning have received very good feedback in trials in recent years. It is believed that in the near future, there will definitely be a mature product that can cross the extremely high technical threshold of the appearance inspection of finished products.
This shows that a mature, usable and reliable machine vision product must require the complementarity of traditional algorithms and deep learning algorithms. For example, the average cycle time in the panel industry can be as short as 2.5s. Under the requirement of high line speed, the algorithm detection time for a single product (calculated according to a data volume of 100Mb) needs to be controlled within 1.5s. At this time, traditional algorithms usually have a speed advantage over deep learning algorithms. On the other hand, for classifications that are difficult to achieve with traditional algorithms and complex scenarios, deep learning algorithms are more likely to show their strengths.
Question: At the "planning/decision-making" and "execution" levels, how should good industrial vision components interact with the overall automation system of industrial manufacturing? What are the more difficult product and solution design problems?
The interaction at the "planning/decision-making" level and the "execution" level can be intuitively understood as the interaction and handshaking between the visual system and the overall machine automation system, or the interaction and handshaking between the upper computer software and the lower computer board/PLC. From the perspective of function division, the visual system theoretically only takes charge of actions related to vision, and the PLC controls all the motion axes, solenoid valves, and sensors of the machine.
Therefore, at the control level, the initialization of the camera/image capture card, the on/off of the light source, the adjustment of the light source brightness, and the storage and detection of images are completely controlled by the visual system. The handling/handover of products, the movement of each axis, the on/off of the air circuit, and the control of air pressure/temperature/safety grating/barcode scanning/emergency stop reset start button are completely controlled by the PLC.
At the interaction level, the communication interaction between the upper and lower computers is required for image acquisition and the feedback of detection results. When the PLC receives the product ID returned by the barcode scanning device, it will send the ID to the upper computer for image naming and storage; when the product moves to the preset image acquisition position, the PLC will notify the upper computer that it can acquire images and wait for the signal that the upper computer has completed the acquisition, and then execute the subsequent process; after the upper computer has completed the image detection, it will feedback the OK/NG result of the product to the PLC, which is convenient for the classification and unloading of products.
When there are a large number of visual workstations in the equipment, the interaction between the upper and lower computers includes the communication between the upper computers of each visual workstation and the communication interaction between each visual workstation and the PLC. Since the action processes of each visual workstation are parallel, and the products at each workstation are not the same (there may be multiple products in the equipment at the same time), the interaction scheme will become very complex.
Question: Innovusion has undertaken many typical industrial vision projects. In these projects, what do you think is the biggest technical challenge?
In industrial vision projects, Innovusion not only provides core software and algorithms but also integrates self-developed or purchased machines and equipment. In this process, the biggest challenge is how the software algorithm team mainly focusing on machine vision and the automation team mainly focusing on optomechatronics can cooperate tacitly. For example, high-precision AOI (Automated Optical Inspection) requires a complete optical scheme, a stable and shock-proof machine, a motion mechanism with high repeat positioning accuracy, and precise linkage between upstream and downstream to match the production line cycle. The software and hardware must be coordinated to achieve the best results.
Another technical challenge is to quickly respond to the introduction of new products to be inspected/measured. There are a variety of products in the industrial industry, and the product iteration speed is fast. Take the electronics manufacturing industry as an example, the switching of machine types requires dozens of operations such as adjusting the product stage, adjusting the position of the vacuum suction nozzle of the handling mechanism, adjusting the working distance of the camera/focusing of the lens/position of the light source, adjusting the image acquisition position, adjusting the position of the probe pressing down, switching the software template, adjusting the detection parameters, optimizing the algorithm model, adjusting the material placement position, etc. Every time the machine type is switched, the machine vision model and the customized part in the overall scheme must quickly adapt to the new scenario.
Question: Innovusion's ManuVision industrial vision platform connects processes such as "perception/understanding", "planning/decision-making", and "execution" to a complete technical platform, which greatly reduces the development and implementation difficulty of industrial vision solutions. From a product perspective, what values can the ManuVision platform contribute to industrial vision scenarios?
The design concept of the ManuVision platform is to make the product development of industrial vision faster and the project delivery of industrial vision lighter. The ManuVision platform includes three key functional modules: Designer, Runtime, and Trainer.
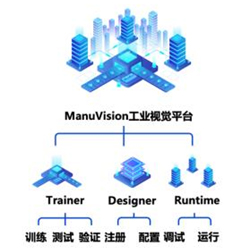
The Runtime module is the device business execution module. Through the interface of this module, one can observe in real time the device production capacity, images of each workstation, detection results, abnormal information, operation logs, etc.; when the device switches to the corresponding product, the corresponding business process and deep learning model can be switched through the Runtime module.
The Designer module is the inspection scheme and business process configuration module. The Designer module encapsulates the core operations in the industrial vision process into functional blocks. By intuitively adding and connecting these functional blocks, the delivery team and the production line equipment engineers of customers can quickly build a complete business process.
The Trainer module has pre-trained models preset. Production line equipment engineers, QCs, and operators do not need any algorithmic foundation. They only need to use the annotation tool to complete the defect annotation, and then the Trainer module can automatically complete the optimization and testing of the preset model, and the model can be deployed with one click on the runtime interface.
Overall, the ManuVision industrial vision platform integrates our overall thinking about industrial vision technologies and products within a unified software framework, and it is an efficient tool for realizing the upgrade from "manufacturing" to "intelligent manufacturing" in industrial vision scenarios. |